AI: Feature or Product?
AI has undergone a meteoric rise, especially since the release of OpenAI's ChatGPT and the rise of large language models (LLMs). These models have had a profound impact on how businesses and individuals approach content creation, problem-solving, and even daily tasks. The sheer power of AI has sparked enthusiasm, with many industries eager to incorporate it into their products and services.
However, we are now at a critical juncture where AI could be overhyped. While the promise of AI revolutionizing industries is immense, we also risk investing in solutions that deliver less value than anticipated. Nevertheless, the potential for AI to trigger a new wave of innovation remains compelling. But one key distinction remains vital: is AI a feature, or is it a product?
AI as a Product
When AI is positioned as a product, it becomes the entire value proposition of the offering. The AI itself is the core product, and its capabilities form the foundation of the value it provides to users. Take OpenAI’s ChatGPT as an example. The product’s value is derived entirely from its ability to generate human-like text, answer complex questions, and provide insights efficiently.
ChatGPT’s impact is measured in terms of productivity gains. When using ChatGPT, users can save substantial time in creating content, summarizing information, or conducting research. By extension then, the key value of AI products lies in their ability to enhance productivity—users can accomplish more, faster, and often with better results.
When developing AI as a product, companies typically follow one of two paths:
First, some companies focus on building AI products based on proprietary and often unique data. This approach can provide a strong competitive advantage because data, especially in regulated or specialized industries, is difficult to replicate. Healthcare AI solutions, for instance, often deal with sensitive patient data and must navigate complex regulatory environments, making their datasets valuable and exclusive.
Another approach is to develop proprietary AI models that become the product's intellectual property. In this case, the advantage lies in the design, training, and optimization of the AI model itself. OpenAI exemplifies this by developing and refining its large language models, which provide unique capabilities that drive user value. The model itself is the core IP and differentiates the product in the market.
Regardless of the development path, the ultimate value proposition of AI as a product remains rooted in productivity gains. These gains can be measured in terms of efficiency, speed, accuracy, or scale, enabling individuals and organizations to achieve more with less effort or time.
AI as a feature
In contrast, when AI is a feature, it plays an enhancement role within a broader product. Here, AI is not the main offering but serves as an incremental value layer that makes the core product more appealing or efficient. The primary product remains independent of AI, but the AI capabilities add a layer of usability, performance, or accessibility.
Consider a database management system (DBMS) that incorporates natural language processing (NLP) for querying data. In a traditional database, users interact using structured query languages like SQL, which require technical knowledge. By integrating an AI-powered NLP feature, users can now ask questions in plain language, making the system more accessible to non-technical users. However, the core product remains the database itself—the AI merely enhances the user experience.
The challenge with “AI as a feature” is in measuring its impact, specifically its productivity gains.
While AI features may deliver initial "wow" moments, it's often difficult to discern whether these features lead to sustained productivity improvements. Does the NLP-based query system truly make data engineers more efficient, or does it only seem impressive initially because it’s a novel way to interact with the system?
In many cases, the "wow" factor from AI innovations can create the perception of enhanced usability, but quantifying these benefits over time can be challenging. Are users actually saving time and achieving more? Or is the AI feature simply providing a more ergonomic but not necessarily more productive interface?
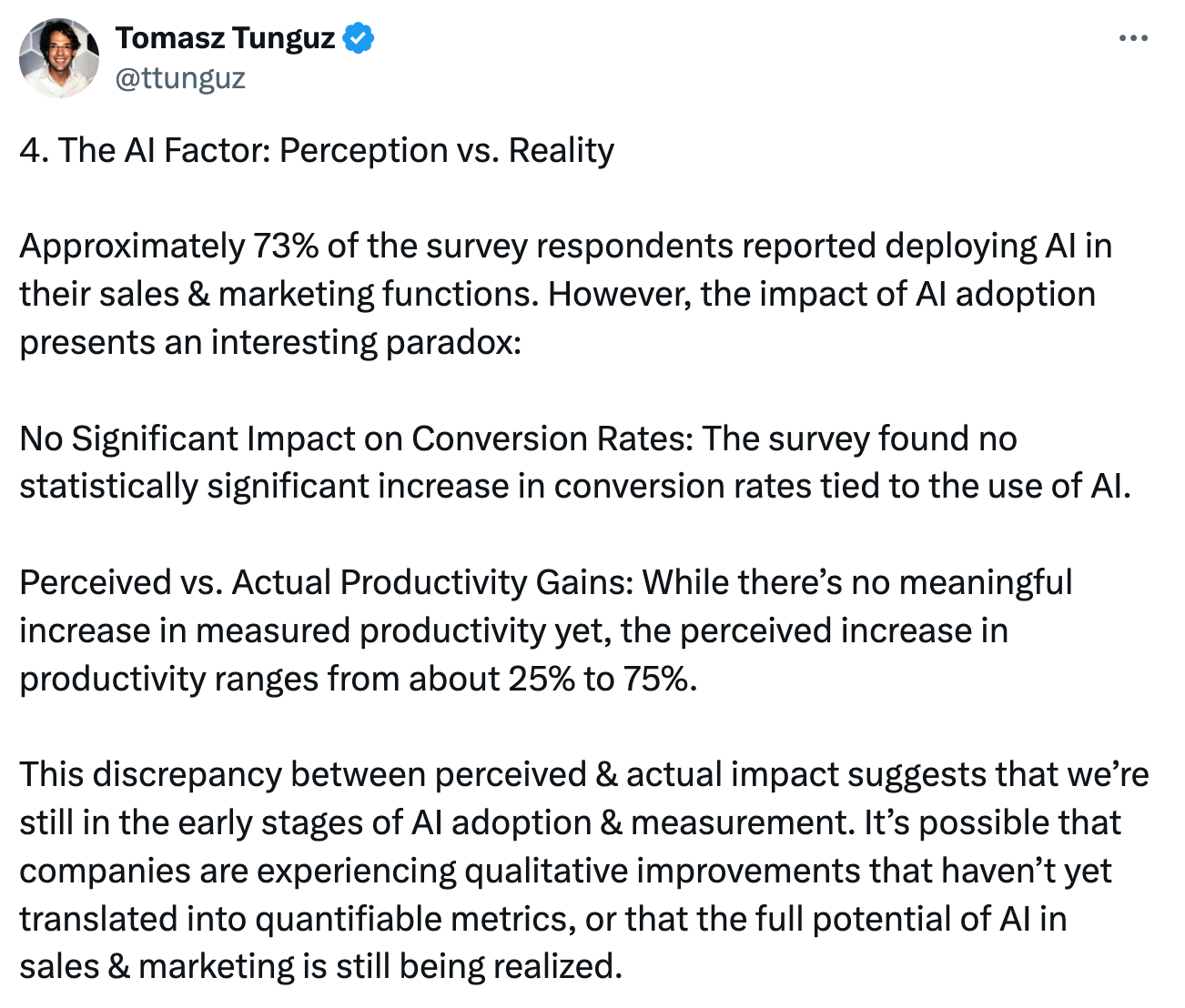
Because of the hype surrounding AI, some companies invest heavily in AI features without clear evidence of the productivity gains they deliver. As a result, we may have seen significant resources poured into AI features that do not meaningfully improve the core product’s value. The risk of over-investing in AI for AI’s sake is real, especially when companies are driven by market pressures rather than clear product strategies. Obviously, if you are Nvidia you are loving all this :)
AI: The Feature + Product
In some cases, AI as the feature evolves to become more important than the core product itself. This phenomenon is particularly relevant in products that generate or manage large amounts of data and that data has a very high noise to signal ratio. AI can be of immense use in this case as it can excel at parsing through vast data sets to extract actionable insights or actions, and in some cases, the value of these insights can outweigh the value of the original product.
This phenomenon of voluminous data that suffers from a high noise to signal ratio is especially common in sectors like security and observability, where data is abundant and real-time insights are critical. Security platforms that monitor vast amounts of system logs, network traffic, or user behavior data can become overwhelming for human operators. AI features within these platforms analyze patterns, detect anomalies, and generate insights far faster than a human could. In such scenarios, the AI feature can save significant time for users, increase productivity, and, in turn, become the primary reason the product is valuable.
In this case, a symbiotic relationship forms between the core product and the AI feature. The core product generates data, which trains and improves the AI model. The AI model, in turn, helps users by providing insights, reducing noise, and identifying valuable signals, saving time and increasing productivity. As users rely more on these AI-driven insights, they increase their usage of the product which in turn generates more data, which feeds back into the system, further improving the AI’s accuracy and usefulness.
This feedback loop drives greater adoption of the product, that could turn the AI feature into a core component that is often more valuable than the original product itself.
In my opinion, category winning products will emerge from two of the three options I mentioned earlier.
The first are companies that are able to provide meaningful productivity, or cost savings, via pure-play AI products will do incredibly well. There are obvious winners today like OpenAI. I think more will emerge that focus on vertical SaaS too, AI can unlock tremendous productivity gains in industries that aren’t entirely digital like healthcare (in particular medical imaging), manufacturing and agriculture. Additionally, AI can unlock productivity gains for most of the G&A functions within an organization.
The second is ones that develop that cyclical relationship between their core product, data and AI. That cycle becomes a competitive moat whilst unlocking tremendous value for customers of these products.
The “losers” in my opinion are companies and products that spread a veneer of AI on their products with no tangible value, likely out of FOMO.